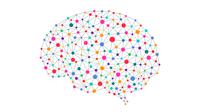
Condensed
matter physics is the study of the collective behavior of infinitely
complex assemblies of electrons, nuclei, magnetic moments, atoms or
qubits. This complexity is reminiscent of the “curse of dimensionality”
commonly encountered in machine learning. Despite this curse, the
machine learning community has developed techniques with remarkable
abilities to classify, characterize and interpret complex sets of data,
such as images and natural language recordings. Here, we show that
modern architectures for supervised learning, such as fully-connected
and convolutional neural networks, can identify phases and phase
transitions in a variety of condensed matter Hamiltonians. Readily
programmable through open-source software libraries, neural networks can
be trained to detect multiple types of order parameter, as well as
highly non-trivial states with no conventional order, directly from raw
state configurations sampled with standard Monte Carlo. Further, Monte
Carlo configurations can be used to train a stochastic variant of a
neural network, called a Restricted Boltzmann Machine (RBM), for use in
unsupervised learning applications. We show how RBMs, once trained, can
be sampled much like a physical Hamiltonian to produce configurations
useful for estimating physical observables. Finally, we explore the
representational power of RBMs, their role in deep learning, and its
possible relationship to the renormalization group.