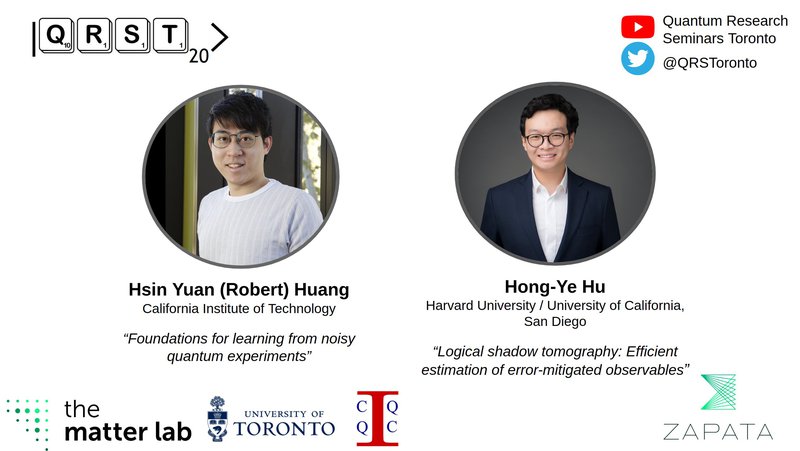
Quantum Research Seminars Toronto consist of two 30 min talks about some Quantum Computation topic. Seminars are given by high-level quantum computing researchers with the focus on disseminating their research among other researchers from this field. We encourage to attend researchers regardless of their experience as well as graduate and undergraduate students with particular interest in this field. Basic notions on quantum computing are assumed, but no expertise in any particular subject of this field.
In this 20th series of seminars, the speakers will be Hsin Yuan (Robert) Huang from California Institute of Technology and Hong-Ye Hu from Harvard University / University of California, San Diego. Their talks are titled "Foundations for learning from noisy quantum experiments" and "Logical shadow tomography: Efficient estimation of error-mitigated observables", respectively.
The event recording, slides and chat history will be published in our Youtube channel and sent to the registered participants.
Looking forward to seeing you all!
___________________________________________________________________
Talk 1:
Title: Foundations for learning from noisy quantum experiments
Abstract:
Understanding what can be learned from experiments is central to scientific progress. In this work, we use a learning-theoretic perspective to study the task of learning physical operations in a quantum machine when all operations (state preparation, dynamics, and measurement) are a priori unknown. We prove that, without any prior knowledge, if one can explore the full quantum state space by composing the operations, then every operation can be learned. When one cannot explore the full state space but all operations are approximately known and noise in Clifford gates is gate-independent, we find an efficient algorithm for learning all operations up to a single unlearnable parameter characterizing the fidelity of the initial state. For learning a noise channel on Clifford gates to a fixed accuracy, our algorithm uses quadratically fewer experiments than previously known protocols. Under more general conditions, the true description of the noise can be unlearnable; for example, we prove that no benchmarking protocol can learn gate-dependent Pauli noise on Clifford+T gates even under perfect state preparation and measurement. Despite not being able to learn the noise, we show that a noisy quantum computer that performs entangled measurements on multiple copies of an unknown state can yield a large advantage in learning properties of the state compared to a noiseless device that measures individual copies and then processes the measurement data using a classical computer. Concretely, we prove that noisy quantum computers with two-qubit gate error rate ϵ can achieve a learning task using N copies of the state, while N^(Ω(1/ϵ)) copies are required classically.
About the speaker:
Hsin-Yuan Huang (Robert) is a Ph.D. student at Caltech advised by John Preskill and Thomas Vidick. His research focuses on understanding how the theory of learning can provide new insights into physics, information, and quantum computing. Some of the central works include classical shadow tomography for learning large-scale quantum systems, provably efficient machine learning algorithms for solving quantum many-body problems and the study of quantum advantages in machine learning.
Talk 2:
Title: Logical shadow tomography: Efficient estimation of error-mitigated observables
Abstract:
Error mitigation is an important task for near-term quantum machines. In this talk, I will introduce a technique to estimate error-mitigated expectation values on noisy quantum computers. Our technique performs shadow tomography on a logical state to produce a memory-efficient classical reconstruction of the noisy density matrix. Using efficient classical post-processing, one can mitigate errors by projecting a general nonlinear function of the noisy density matrix into the codespace. The subspace expansion and virtual distillation can be viewed as special cases of the new framework. In addition, I will show our method is favorable in the quantum and classical resources overhead.
Last but not the least, I will conclude my talk on our newly proposed shadow tomography scheme that only requires local invariance of the gate set. The new scheme sheds light on the near-term implementation of entanglement assisted randomized measurements.
About the speaker:
Hong-Ye Hu is an incoming HQI Fellow at Harvard starting Fall 2022, and he is currently a consultant at QuEra Computing. Prior to that, he was an intern with the NASA Quantum AI team and Salk Institute. He got his Ph.D. from University of California San Diego in 2022, and his B.S from Peking University in 2016.